AI Art Weekly #47
Hello there, my fellow dreamers, and welcome to issue #47 of AI Art Weekly! 👋
Between vision of multi-agent civilization sims running on GPT-5 and the first iteration of the Terminator this week, I’ve yet another packed issue of amazing developments from the world of AI for you. Let’s jump in! These are this weeks highlights:
- DragNUWA: Microsoft’s new video generation model
- CoDeF: A new approach to temporal consistent video to video generation
- IP-Adapter: A new image prompt adapter for text-to-image models
- Inst-Inpaint: Hands free object removal from images
- Unimask-M: Inpainting for human motion
- CLE Diffusion: Image segmentation meets light enhancement
- Semantics2Hands: Transferring Hand Motion Semantics between Avatars
- Interview with AI artist Le Moon
- Fooocus: A merge between Stable Diffusion and Midjourney
- and more tutorials, tools and gems!
As a Pro Member, you’ll be backing the evolution and expansion of AI Art Weekly. Together we’re at 41/100% of our Twitter API milestone. Join us!
Cover Challenge 🎨
I went to my second Heilung ritual last week and felt inspired to visit the world of pagan culture for this weeks theme. The reward is $50 and the Challenge Winner role within our Discord community. This rare role earns you the exclusive right to cast a vote in the selection of future winners. Rulebook can be found here and images can be submitted here. I’m looking forward to your submissions 🙏
News & Papers
DragNUWA
One of the main things that’s lacking in current video generation models is control. Microsoft’s DragNUWA is trying to solve that by utilizing text, images, and trajectory as three essential factors to facilitate highly controllable video generation. Can’t wait for the time when we get real time diffusion and can just drag and drop our videos together.
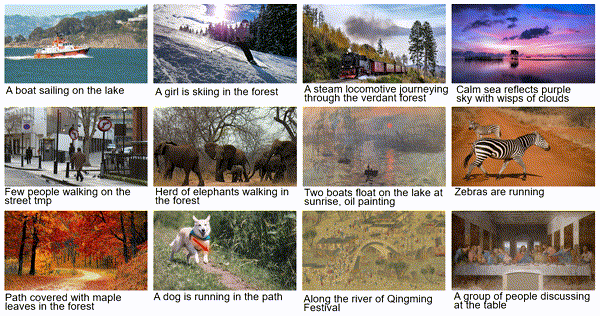
DragNUWA examples
CoDeF: Content Deformation Fields for Temporally Consistent Video Processing
But not only text and image-to-video is getting better by the week, also video-to-video has seen improvements. One of the biggest issues has always been temporal consistency. CoDeF tries to solve that with a new approach consisting of a canonical content field aggregating the static contents in the entire video and a temporal deformation field recording the transformations from the canonical image to each individual frame along the time axis. This also opens up new possibilities like point-based tracking, segmentation-based tracking and video super-resolution.
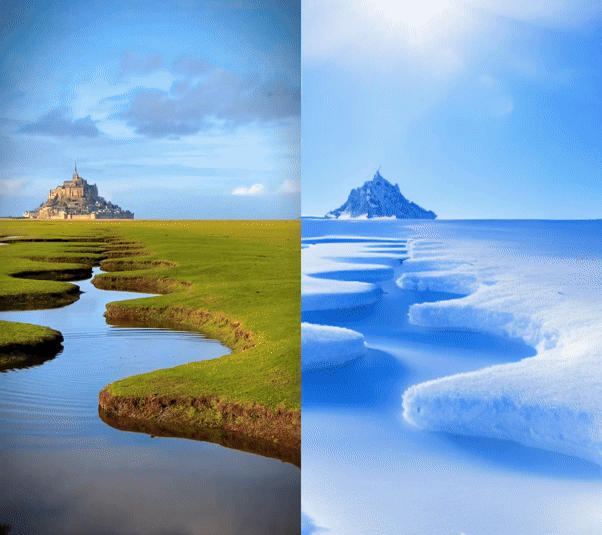
CoDeF example
IP-Adapter: Text Compatible Image Prompt Adapter for Text-to-Image Diffusion Models
Similar to ControlNet and Composer, IP-Adapter is a mutli-modal guidance adapter for image prompts which works with Stable Diffusion models trained on the same base model. The results look amazing.
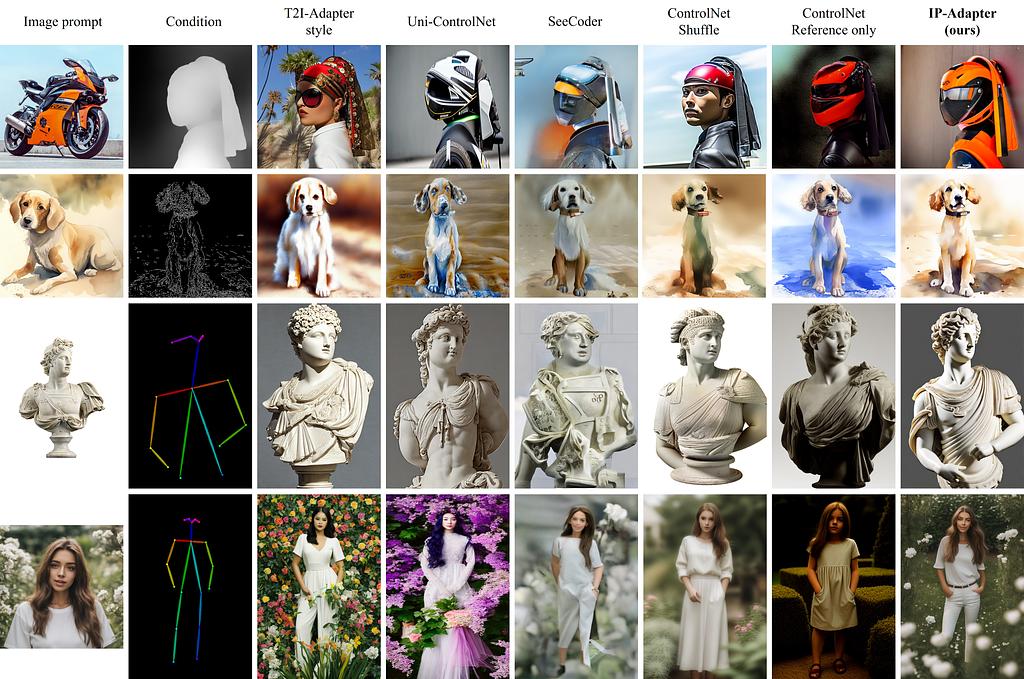
IP-Adapter comparison with other methods
Inst-Inpaint: Instructing to Remove Objects with Diffusion Models
The days in which you have to select the objects you want to remove in Photoshop might be gone soon. Inst-Inpaint enables the use of natural language to remove objects from images.
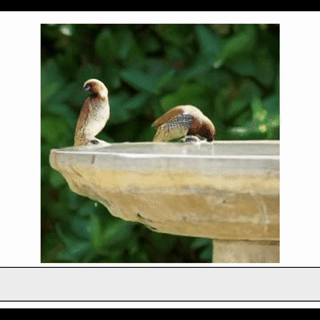
Inst-Inpaint examples
MASK-M: A Unified Masked Autoencoder with Patchified Skeletons for Motion Synthesis
Two thoughts when I look at Unimask-M: 1) Terminator will be able to predict our movements soon and 2) This will make creating animation so much more efficent. Completion makes it possible to only draw some parts of the body and complete the rest automatically. In-betweening makes it possible to generate all in-between keyframes with a few starting and end frames. Exciting times.
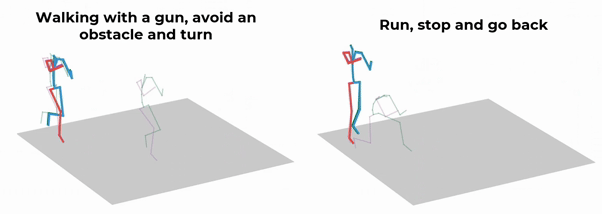
Unimask-M examples. Now you know why I thought of 1) above 😅
CLE Diffusion: Controllable Light Enhancement Diffusion Model(MM 2023)
So far light enhancements on images was either a one size fits all solution or a manual and labour intense task. CLE Diffusion tries to change that by incorporating SAM (Segment-Anything Model) – this allows users to just click on objects to specify the regions they wish to enhance.
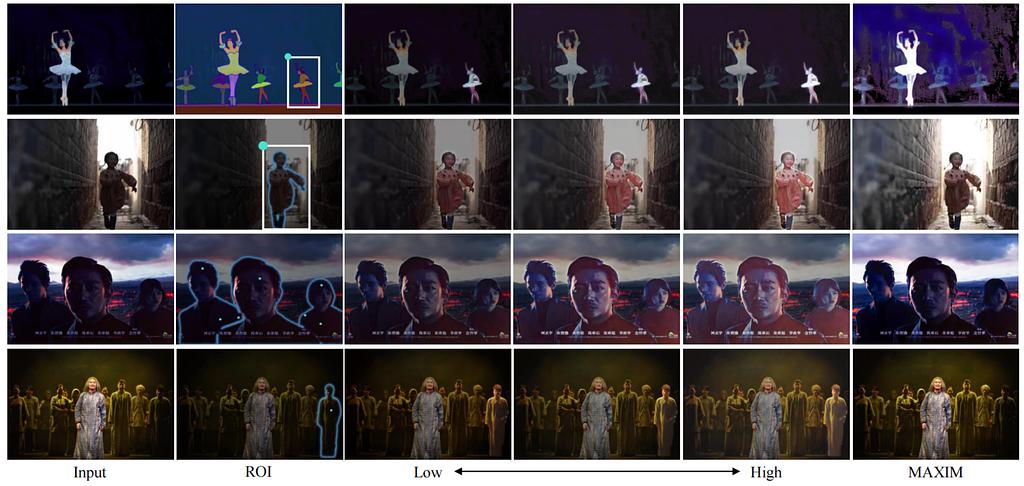
CLE Diffusion examples
Semantics2Hands: Transferring Hand Motion Semantics between Avatars
AI artists know: hands are hard. While Semantics2Hands will not help us with generating images, it might help us one day with animating them. Given a source hand motion and a target hand model, the method can retarget realistic hand motions with high fidelity to the target while preserving intricate motion semantics.
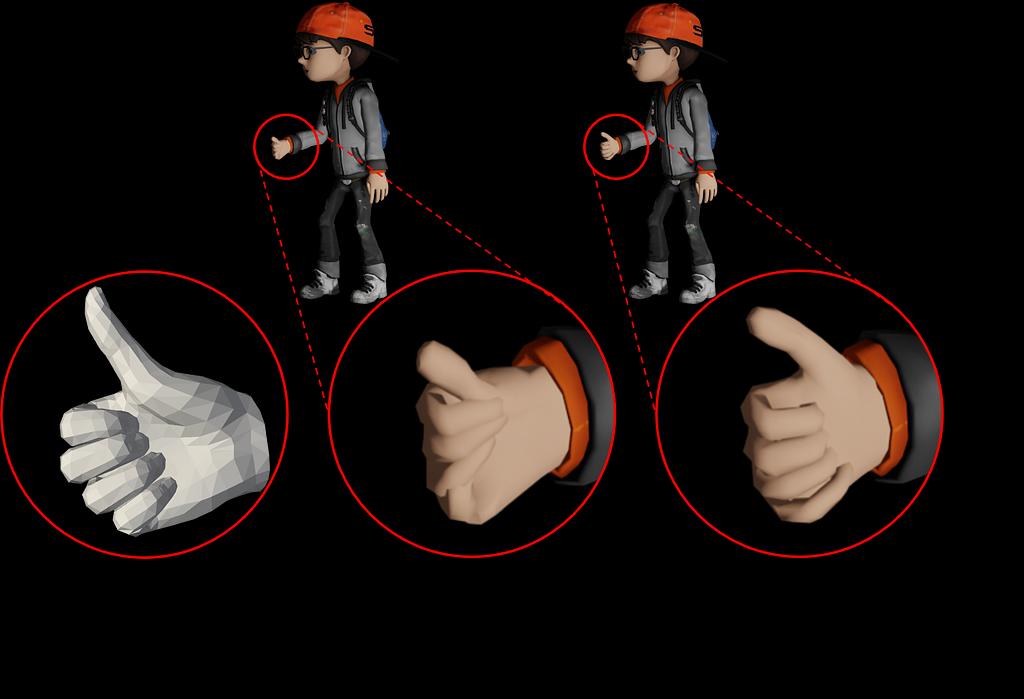
Despite the accurate body motions, errors introduced by copying finger joint rotations make the “thumb-up” gesture illegible.
More papers & gems
- Relightable Avatar: Relightable and Animatable Neural Avatar from Sparse-View Video
- RIGID: Recurrent GAN Inversion and Editing of Real Face Videos
- DeDoDe: Detect, Don’t Describe / Describe, Don’t Detect for Local Feature Matching
- Color-NeuS: Reconstructing Neural Implicit Surfaces with Color
- NCP: Neural Categorical Priors for Physics-Based Character Control
@FARRAHXYZ together with @artandvault published the first AIMAGINEZ issue which I was honoured to be a part of! Dive into the artist’s narratives, their unique journeys, & the captivating visions they share (including mine 😉).
Using the power of AI, this SuperRare exhibit curated by @Historic_Crypto features thirty alternate timelines that will leave you questioning everything you thought you knew about history…
@ai_filmmaker put together an AI animated Studio Ghibli tribute animation with Midjourney and After Effects into which @blacktraced infused rotoscoped characters with RunwayML.
AI Town is a virtual town where AI characters live, chat and socialize. The code is open-source and people already started to create their own versions like Cat Town.
Interviews
This week I had the pleasure to interview French based AI artist Melody Bossan aka Le Moon. Le Moon’s background is in TV production and video game marketing. Her work expresses her memories, fears and dreams in a nostalgic and disturbing style and explores themes of horror, weirdness, and fever dream parallel realities through an approach that she calls absurd realism. Enjoy!
Tools & Tutorials
These are some of the most interesting resources I’ve come across this week.
Stable Diffusion shines through being offline, open source, and free while Midjourney is loved for it’s ability to produce stunning images with a simple prompt. Fooocus aims to combine the best of those two worlds into one.
LoRA the Explorer is a HuggingFace space by @multimodalart that lets explore and test SDXL LoRAs without having to dodge semi-naked waifus.
ResMem is a tool to calculate the memorability of an image. The model is already three years old though, but might be a helpful tool if you’re unsure which image to select from a range of images. The code can be found on GitHub.
If last weeks AutoTrain Dreambooth notebook wasn’t of help with training an SDXL model, this video might be. It’s a step by step guide on how to train a Stable Diffusion XL model.
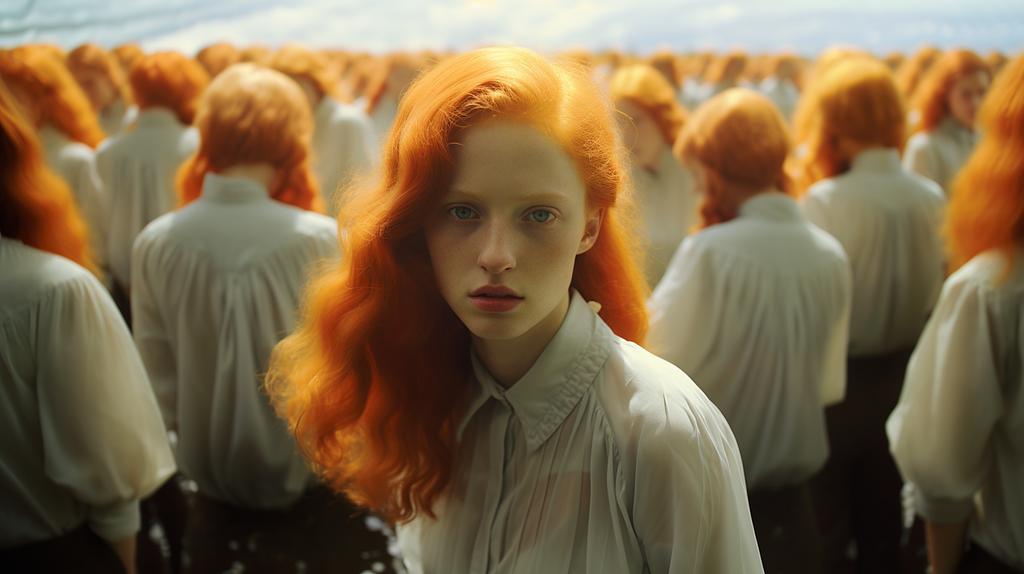
“Visions of Endless Echoes” by me
And that my fellow dreamers, concludes yet another AI Art weekly issue. Please consider supporting this newsletter by:
- Sharing it 🙏❤️
- Following me on Twitter: @dreamingtulpa
- Buying me a coffee (I could seriously use it, putting these issues together takes me 8-12 hours every Friday 😅)
Reply to this email if you have any feedback or ideas for this newsletter.
Thanks for reading and talk to you next week!
– dreamingtulpa